AI at the Crossroads: Balancing Innovation and Security in Multi-Cloud Environments

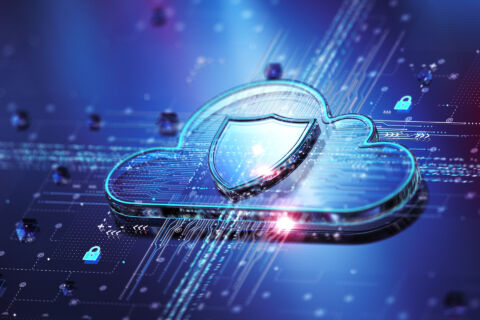
As organizations continue to prioritize seamless efficiency and productivity gains, AI is proving an indispensable tool for optimized workflows. Unsurprisingly, this has created a plethora of platforms and services that organizations can choose from to maximize the value of their AI investments. One key trend is the rise in agentic AI. According to Gartner’s Top Technology Trends for 2025, 33% of enterprise software applications will include agentic AI capabilities this year.
In February, Salesforce and Google announced that they would be expanding their partnership with the integration of Gemini, Google’s advanced AI assistant, into Agentforce, which promises several key benefits:
- Enhanced multi-modal capabilities. Agents can handle images, audio, and video, performing complex tasks with real-time insights.
- Improved customer engagement. Features like real-time voice translation, intelligent handoffs, and personalized recommendations enhance customer interactions.
- Unified platform on Google Cloud. Running Salesforce apps on Google Cloud offers secure infrastructure, new regional access, and simplified procurement.
- Flexibility and choice. Businesses can tailor AI solutions using various models, avoiding lock-in to a single provider.
- Increased efficiency. Advanced processing capabilities ensure faster responses and reduce operational costs.
While these benefits highlight the transformative potential of the Gemini-Agentforce integration, they also underscore a critical prerequisite for successful AI implementation. Beyond the excitement of cutting-edge capabilities lies a fundamental challenge that organizations must address — comprehensive data security.
Security Risks in Multi-Cloud AI Deployments
With 74% of cloud buyers opting for multi-cloud environments as reported by IDC, it’s no wonder that security remains a top priority for organizations when leveraging AI, alongside a strong reliance on multiple cloud providers. According to Gartner, worldwide end-user spending on public cloud services will reach $723.4 billion by 2025. More importantly, this growth is the result of AI systems “unabatedly accelerating the role of cloud computing” in today’s workflows.
While Gemini’s integration in Agentforce offers important infrastructure security features such as end-to-end encryption, multi-factor authentication (MFA), and regular security audits, it’s important to note that the continuous innovation in AI systems will lead to an increasing number of similar partnerships and developments in agentic AI that promise to deliver an intelligent data foundation.
In addition, organizations will increasingly leverage more AI models and agents to address market trends, customer behavior, and the continuous demand for innovation. In doing so, organizations will not only need to manage the settings and configurations of these agents but also control the access to any underlying organizational data.
It’s important to note that dedicated AI agents will specialize in assigned roles; some will specialize in sales, some in marketing, and others in support. Therefore, ensuring that agents have access to data relevant to their specific roles is paramount for quality output. However, organizations must also evaluate which users are utilizing these agents, as well as where the outputs will be consumed (internally, with partners, with customers, or publicly), since these agents have access to potentially sensitive data.
Considering the ceaseless nature of advancements in AI, one thing remains constant: Organizations must keep in mind that an intelligent data foundation is not possible without first establishing one that is built on quality and secure content.
In their pursuit to realize the value of various AI options available to their organization, business leaders must therefore pay attention to the following security considerations:
Ambiguity in the AI Shared Responsibility Model
The shared responsibility model is a framework that distinguishes between the security and compliance responsibilities of cloud service providers (CSPs) and their customers. In this case, the integration of Gemini across multiple cloud environments creates complexities in the shared responsibility framework, as organizations may face challenges in determining who is responsible for securing AI training data, models, and outputs at each stage of the AI workflow. When these obligations are unclear, security gaps can emerge, increasing the risk of data breaches and unauthorized access.
With the cost of a data breach averaging $4.88 million in damages in 2024, there’s no question that organizations must clearly delineate and communicate the roles and responsibilities that fall under their internal team and those that fall under CSPs. In addition to the possibility of breaches, the lack of such delineation may also lead to compliance violations. To avoid these, organizations must draw up clear contracts and service level agreements (SLAs) to outline not just their duties as customers but also the expectations for each party involved.
AI-Specific Access Control Complexities
Managing access control for enterprise AI involves setting granular permissions to determine which personnel can train models, view AI insights, or modify AI configurations. However, this complexity may be heightened when organizations deal with multi-cloud environments due to the varying access control mechanisms in each platform. In addition to data leaks, potential misuse of AI capabilities can also arise. To ensure consistent and secure access control, organizations must implement robust identity and access management (IAM) frameworks that span all cloud environments. Reducing data breaches and compromised AI models also entail regularly reviewing and updating access permissions and employing automated tools to monitor and enforce access policies.
Data Flow Risks Across Environments
When data moves across different cloud environments, this can introduce specific risks, such as data interception, loss, or corruption. These risks are exacerbated by the varying security protocols and standards across platforms. In the context of AI, the complexity increases as AI models require large datasets that are transferred and processed across multiple environments. This data sprawl can lead to vulnerabilities such as data leaks, where sensitive information might be exposed during transfer. Additionally, AI models themselves can be targeted for data poisoning attacks, where malicious actors manipulate the data to skew the model's outputs. These risks underscore the role of data security posture management (DSPM) for organizations to execute a proactive approach to data protection.
DSPM is a cybersecurity practice that identifies sensitive data across cloud environments, assesses vulnerabilities, and automates compliance monitoring. DSPM provides organizations with visibility over where sensitive data resides, how it moves, who accesses it, and what security risks exist within their data infrastructure. By implementing DSPM, organizations gain a comprehensive understanding of their security posture, allowing them to preemptively address vulnerabilities and quickly resolve data security issues before exploitation can occur. While data protection and backup remain critical to restoring data integrity, organizations without DSPM will struggle to control what individuals can put in their environments, including content designed to poison their data. It is also equally important that organizations control who can download, delete, modify, as well as add, create, or upload data.
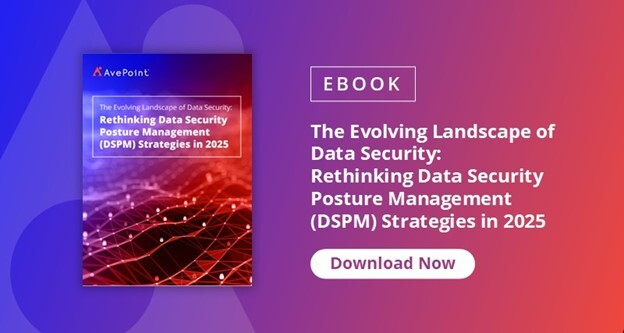
By leveraging a proactive stance in security, organizations can ensure data integrity and security across diverse environments — critical areas to maintain the reliability and trustworthiness of AI-driven processes. AI systems are non-negotiable in today’s workforce as they open the doors to unparalleled productivity gains and informed decision-making. These security considerations, rather than roadblocks, are prime opportunities for organizations to enhance their data management before they invest in their AI system of choice.
Strategic Protection for Leveraging AI
To ensure that they have the proper security guardrails in place, organizations must implement the following measures:
Cloud Security Posture Management (CSPM)
While DSPM focuses on identifying and protecting sensitive data across cloud environments, CSPM is geared toward maintaining the overall security posture of cloud infrastructures by continuously assessing and remediating risks. CSPM tools are essential for identifying and remediating risks across cloud infrastructures, including infrastructure-as-a-service (IaaS), software-as-a-service (SaaS), and platform-as-a-service (PaaS). These tools continuously assess the security posture of cloud environments by maintaining an up-to-date inventory of assets and configurations. CSPM solutions provide detailed visibility into the security state of resources and offer guidance for fortifying an organization’s security posture. These solutions detect misconfigurations, compliance violations, and potential vulnerabilities, ensuring that security policies are consistently enforced across multi-cloud environments. By automating the assessment and remediation processes, CSPM tools reduce the risk of human error and enhance overall cloud security.
Unified Identity and Access Management (IAM) Frameworks
Unified IAM frameworks are critical for managing user identities and access permissions across all cloud environments. These frameworks integrate various identity management components, such as access management (AM), privileged access management (PAM), and identity governance and administration (IGA) into a cohesive system. By providing a centralized platform for identity management, IAM frameworks ensure that the right users have the right access to resources while preventing unauthorized access. They support single sign-on (SSO), MFA, and role-based access control (RBAC), enhancing security and user experience. Unified IAM frameworks also streamline compliance efforts by providing comprehensive audit trails and access reviews.
Automated Security Monitoring and Incident Response
When security monitoring and incident response are automated, they deliver continuous visibility and rapid response capabilities across the entire multi-cloud ecosystem. These tools use advanced algorithms and machine learning to detect anomalies, potential threats, and security incidents in real-time.
By automating repetitive tasks, such as log analysis and threat detection, these solutions enable security teams to focus on more strategic activities. Automated incident response platforms can quickly contain threats and mitigate risks, reducing the impact of security breaches. They also provide centralized dashboards for reporting and managing security alerts, ensuring that organizations can maintain a robust security posture and respond effectively to evolving threats.
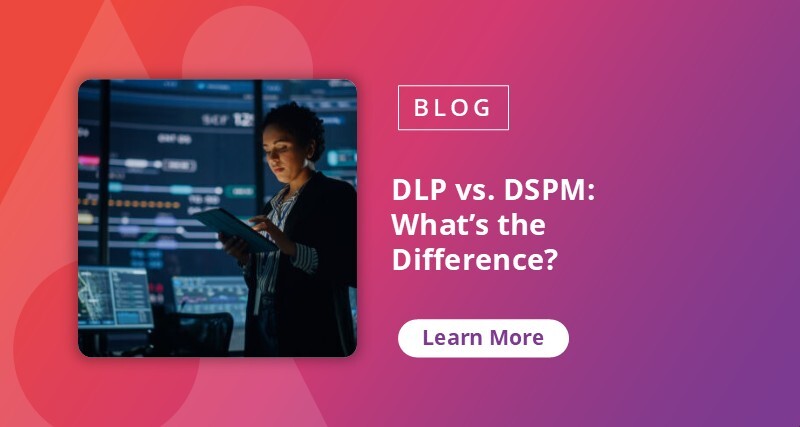
Data Loss Prevention (DLP) Solutions
DLP solutions are designed to protect sensitive data from unauthorized access, misuse, or exfiltration. These solutions enforce security policies based on the content and context of data, whether it is in use, in motion, or at rest. DLP tools monitor data flows across the organization and apply consistent policies regardless of where the data resides (such as on-premises or in the cloud). By detecting and blocking unauthorized data transfers, ensuring compliance with regulatory requirements, and protecting intellectual property, these tools help safeguard organizations from data breaches. DLP solutions also provide visibility into data usage patterns, enabling organizations to identify and mitigate potential insider threats and data loss incidents.
To enhance resilience, organizations can integrate DLP with robust backup and disaster recovery systems. These systems ensure data integrity by providing automated backups, granular restores, and allowing for swift recovery in case of data loss or corruption.
Building a Resilient AI Foundation
The integration of Gemini into Agentforce demonstrates the transformative potential of AI in enhancing organizational productivity and operational efficiency. However, as businesses embrace these technological advancements, addressing security challenges in multi-cloud environments becomes critical. A holistic security solution is essential, encompassing advanced encryption, real-time monitoring, and clear responsibility delineation between internal teams and CSPs.
By establishing a robust data foundation, organizations can confidently navigate the complex landscape of AI technologies. This proactive approach not only mitigates risks but also enables sustainable innovation, ultimately driving long-term growth and operational efficiency.
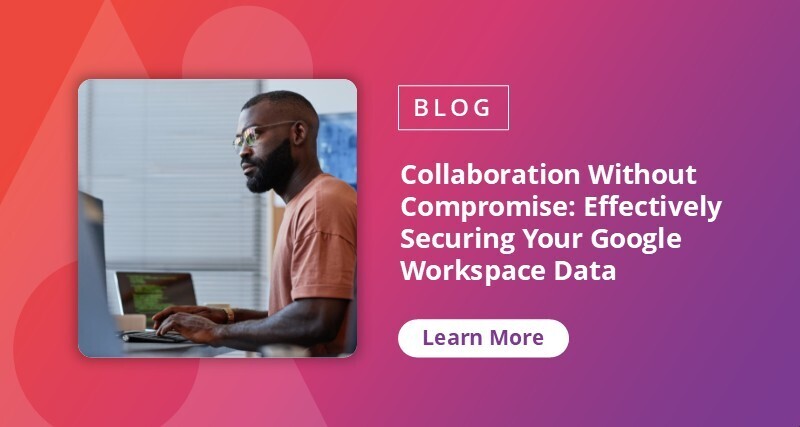

Ava Ragonese is a Product Marketing Manager at AvePoint, leading the GTM of data security solutions for Google Workspace and Cloud. She helps organizations focus on quality data and insights to drive innovation and how multi-cloud collaboration can impact businesses. Ava has a M.Eng. in Systems Analytics from Stevens Institute of Technology and enjoys bringing her technical acumen to complex business decisions such as AI adoption.